Artificial Intelligence (AI) has transitioned from a futuristic notion to a core element of our daily existence, revolutionizing industries and altering business operations fundamentally. AI’s capabilities, from chatbots enhancing customer service to predictive algorithms forecasting market movements, are setting the stage for it to become an essential tool across various sectors.
However, the journey to effectively incorporate AI presents unique challenges for both startups and established enterprises. Recognizing the undeniable advantages, it’s equally important to understand and navigate the limitations of contemporary AI technologies for fruitful integration.
This blog post will examine five principal limitations of AI and offer strategies to maximize its benefits for your business pursuits.
Limitations of AI Implementation in Business
A crucial step in successfully using AI is understanding the advancements in deep learning – a type of AI that improves the accuracy of predictions and classifications through large neural networks that mimic the human brain.
The most common networks, convolutional neural networks (CNNs) and recurrent neural networks (RNNs), improve their accuracy by learning from data and using backpropagation algorithms. Despite progress, deep learning and AI face challenges that need more development.
One key challenge is customizing AI strategies to fit specific problems and data availability. These systems need large amounts of labeled data to perform complex tasks effectively. Gathering and labeling such data can be difficult and labor-intensive.
Moreover, the opacity of deep learning models, or their “black box” nature, adds complexity. This is problematic in situations where understanding an algorithm’s reasoning is crucial, like legal or regulatory decisions. The need for transparency in AI’s decision-making processes is becoming more evident as AI continues to impact society and individual lives.
In summary, this discussion outlines five key limitations of AI and the ongoing efforts to address these challenges, marking a significant step in integrating artificial intelligence into business strategies more broadly.
AI Limitation 1: Data Labeling Bottleneck
The Limitation:
Supervised learning, the cornerstone of training modern AI models, demands an enormous amount of human effort to label and categorize data accurately. This method is not only costly and prone to errors but also time-consuming.
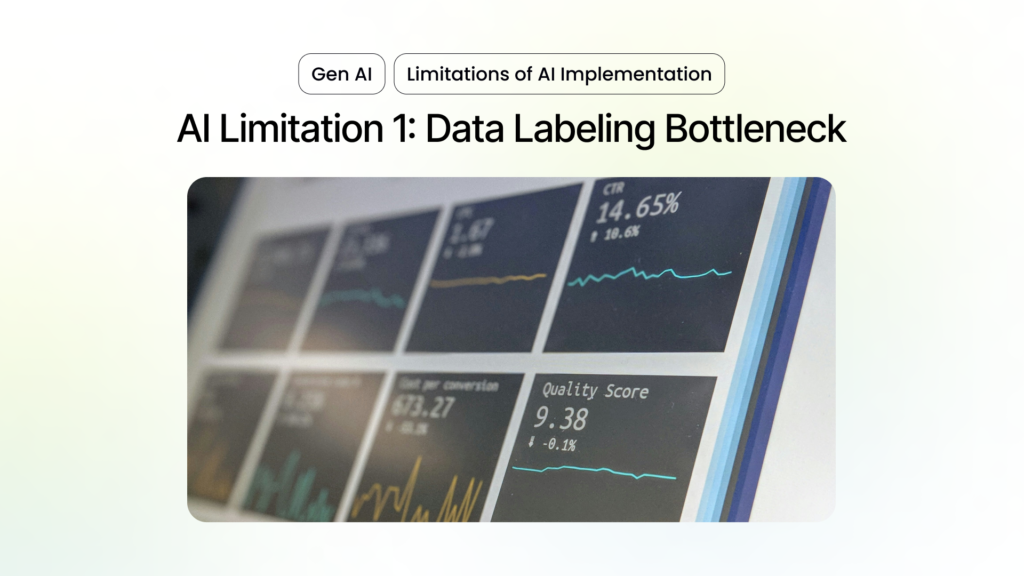
For instance, to train autonomous vehicle systems, companies must hire numerous individuals to manually annotate video footage from prototype vehicles. Despite its widespread use, the drawbacks of supervised learning have spurred the search for more efficient training methods.
The Alternative Approach:
In-Stream Supervision: Spearheaded by Eric Horvitz and his team at Microsoft Research, this innovative method involves labeling data during its natural usage, significantly reducing the need for manual annotation.
Unsupervised and Semisupervised Learning: These strategies lessen the reliance on extensive, human-labeled datasets. Noteworthy among them are:
- Reinforcement Learning: This paradigm shifts towards unsupervised learning, allowing algorithms to learn through trial and error. It uses rewards and penalties to encourage correct actions, leading to achievements that can surpass human capabilities. Its application ranges from mastering games to optimizing decisions in finance and logistics, encouraging the discovery of strategies that may be beyond human insight.
- Generative Adversarial Networks (GANs): Employing a semisupervised technique, GANs involve two networks in a competitive process to refine data understanding. This approach helps in reducing the dependence on human-labeled data by creating realistic data samples for training. GANs are promising in various fields, such as art creation and medical diagnostics, by offering detailed data analysis capabilities.
The limitations of supervised learning have catalyzed the development of alternative techniques like reinforcement learning and GANs. These methods reduce the necessity for human data labeling and advance AI’s learning and adaptation capacity in complex scenarios, marking pivotal strides toward more autonomous and effective AI systems for the future.
AI Limitation 2: Massive Training Data Dilemma
The Limitation: Recent advancements in AI and machine learning, especially with simple linear models, can match professionals’ expertise in fields like medicine. However, these technologies require large, comprehensive labeled datasets. Deep learning methods, for example, need thousands to millions of data records for accuracy comparable to humans, creating a significant barrier to progress.
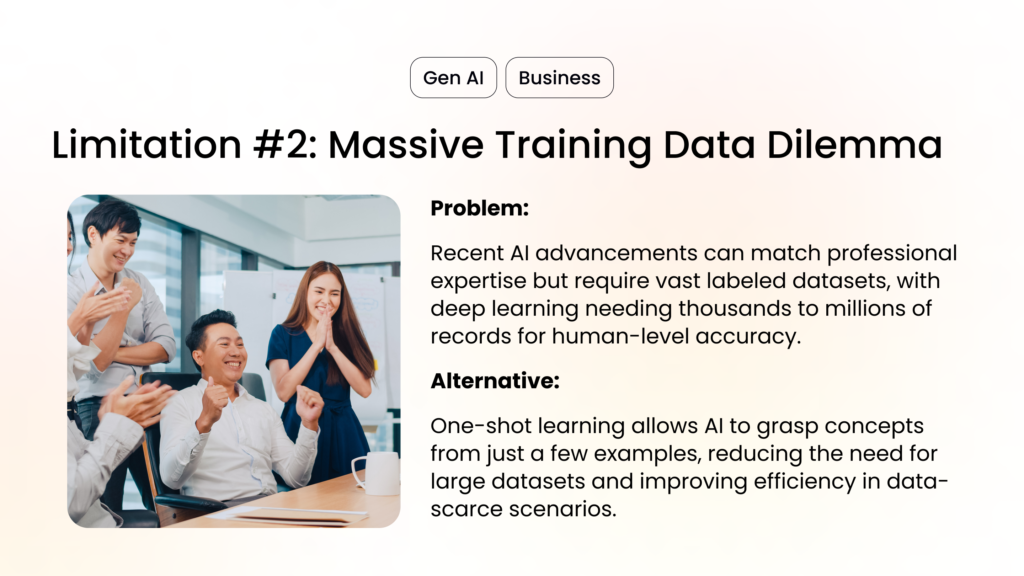
The Alternative Approach: One-shot learning offers a revolutionary technique that reduces the need for large datasets by enabling an AI model to understand a concept from just a few real-world examples, sometimes even one. This method brings AI closer to human learning efficiency and is particularly useful in scenarios where data acquisition is challenging. It represents a promising solution to overcoming the large dataset requirement in machine learning.
AI Limitation 3: The Explainability Issue
The Limitation: Explainability has always been a challenging aspect of AI systems, but the issue has become more prominent with the rise of deep learning. These sophisticated models, while enabling a plethora of advanced applications, introduce a level of opaqueness that makes it difficult to understand their decision-making processes.
The complexity and size of these models exacerbate the problem, making it challenging to articulate why a specific decision was made, especially in real-time scenarios.
This lack of transparency is a significant barrier to the adoption of AI in fields where understanding AI’s “thought process” is crucial or even mandated. Moreover, as AI applications broaden, regulatory pressures for explainable AI are expected to increase, highlighting the urgent need for solutions that can demystify AI decisions.
The Alternative Approach: In response to the growing demand for transparency in AI, two emerging approaches show considerable promise: Local Interpretable Model-Agnostic Explanations (LIME) and attention methods. LIME works by dissecting which aspects of the input data are most influential in a model’s decision-making process. This involves segmenting the data and monitoring how changes in specific segments affect predictions.
Through this observation, it’s possible to create a proxy model that offers a more nuanced interpretation of the AI’s decision-making (for instance, determining facial recognition based on the presence or absence of specific features like eyes or noses).
On the other hand, attention techniques aim to shed light on the decision-making process by highlighting parts of the input data deemed most crucial by the model for making a particular decision. This approach is akin to focusing on a mouth to discern whether an image portrays a human being.
Both methods represent significant strides toward enhancing the transparency of AI models, thereby increasing their trustworthiness and facilitating wider acceptance in sectors where explainability is not just beneficial but necessary.
AI Limitation 4: Generalizability Gap
The Limitation: Unlike humans, AI models find it challenging to transfer their learning from one scenario to another. Achievements of a model in one context tend to remain confined to that specific context, leading to a repetitive cycle of resource investment for training new models for similar tasks.
The Alternative Approach: Transfer learning emerges as a promising strategy, enabling AI models to leverage knowledge from one task to apply it to similar yet distinct tasks, significantly cutting down the time and resources needed for developing new AI applications.
DeepMind’s success with transfer learning in simulation-to-real-world applications exemplifies its potential. Additionally, meta-learning offers an avenue for automatizing the design of machine-learning models, promising to accelerate the development of AI tools and applications, potentially surpassing human capabilities in the process.
Problem 5: Amplification of Biases
The Limitation: Bias in AI is a unique issue that goes beyond technical hurdles. Human biases, whether conscious or not, can influence the selection of data, leading to potentially severe social consequences.
These biases can skew AI models through uneven data collection practices and analysis, resulting in issues ranging from unfair recruiting practices to skewed legal judgments.
Often, these biases remain hidden, cloaked under the guise of advanced data science or proprietary algorithms, and can lead to distorted outcomes in various fields.
The Alternative Approach: Addressing AI bias requires a sophisticated approach that combines a deep understanding of data science with an awareness of societal dynamics.
Efforts are now underway in academic, nonprofit, and private sectors to identify and implement best practices for removing bias from AI. This involves not only technical solutions but also a broader comprehension of how social forces shape data collection and interpretation.
As AI technology evolves and is applied in new areas, the challenge of debiasing becomes increasingly complex, necessitating a commitment to continuous research and ethical vigilance to ensure AI applications are fair and equitable.
Harnessing AI for Your Business
The journey towards comprehensive AI integration is fraught with challenges but also offers immense untapped potential. Achieving widespread commercial use of AI advances might take years, yet the vast possibilities it presents indicate that imagination may be our biggest limitation. Here are strategies for leaders aiming to stay ahead:
- Stay Educated and Informed: Understanding the capabilities of contemporary AI tools and anticipating future advancements is crucial. Engage with data scientists, consult AI pioneers, and participate in AI conferences to gain accurate insights. Resources like the AI Index offer valuable ongoing updates.
- Develop a Sophisticated Data Strategy: Effective AI algorithms require a solid foundation of data. Crafting a comprehensive data strategy that encompasses technology integration, data availability, labeling, and governance is vital. Even as new techniques aim to reduce data needs, a robust understanding and utilization of your data points are essential.
- Leverage Lateral Thinking: Explore how AI solutions can be applied across different business challenges and sectors. Encourage the sharing of insights across business units to discover versatile applications of AI technologies.
- Innovate and Pioneer: Staying updated with current AI technologies isn’t enough. Collaborate with experts to explore high-impact use cases using emerging techniques. Utilize open-source tools, datasets, and pre-trained models to maintain a competitive edge and possibly secure a first-mover advantage.
Embracing these strategies can help leaders navigate the complexities of AI integration while unlocking its full potential for their businesses. However, it’s also crucial for leaders to consider the ethical implications of AI and ensure responsible use of these technologies.
By staying informed, fostering lateral thinking, and continuously innovating, leaders can confidently embrace AI as a powerful tool for driving their organizations toward success in the digital age. The future is here, and those who boldly embrace it will be the ones leading the way into a new era of business.