Financial institutions are increasingly using artificial intelligence (AI) to automate tasks, improve efficiency, and personalize services. In fact, the finance sector exhibits one of the highest adoption rates of AI across industries. This trend is also adopted by the largest banks in the Americas and Europe, such as Captial One, JPMorgan Chase, and the Royal Bank of Canada.
While AI offers significant advantages, ethical considerations surrounding bias and fairness require careful attention. The potential consequences of bias in AI extend beyond fairness concerns. Unethical AI practices could erode trust in financial institutions, a crucial element for overall financial stability.
By acknowledging these challenges, we can work towards ensuring that AI is used responsibly and ethically within the financial sector. As AI adoption continues to grow, regulators and institutions alike will need to develop strategies to mitigate bias and safeguard financial stability.
This blog post explores the ethical considerations and bias issues associated with AI in finance and banking, providing insights and strategies for mitigating these challenges to ensure fair and transparent AI systems.
Ethical Concerns in AI Deployment
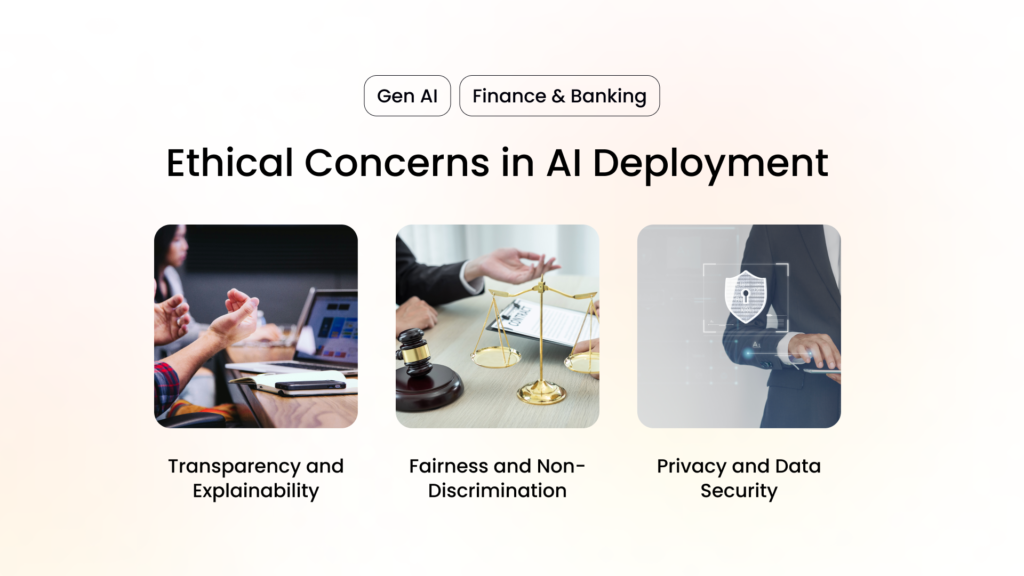
1. Transparency and Explainability
One of the primary ethical concerns in AI deployment is the transparency and explainability of AI models. Financial institutions must ensure that their AI-driven decisions are transparent and understandable to both regulators and customers. However, achieving this is challenging because AI algorithms, especially those based on deep learning, often operate as “black boxes” with complex and opaque decision-making processes.
Importance of Transparent AI Models
Transparent AI models are crucial for maintaining consumer trust and regulatory compliance. When customers understand how decisions are made, they are more likely to trust the institution and feel confident in the fairness of the outcomes.
Challenges in Making AI Decisions Explainable
The complexity of AI models can make it difficult to provide clear explanations for specific decisions. Financial institutions need to invest in developing explainable AI (XAI) techniques that can demystify the decision-making process without compromising the model’s accuracy and effectiveness.
2. Fairness and Non-Discrimination
Ensuring that AI systems do not perpetuate or exacerbate biases is another critical ethical concern. AI algorithms can inadvertently reinforce existing biases present in historical data, leading to unfair and discriminatory outcomes.
Examples of Biased Outcomes in Credit Scoring and Loan Approvals
- Credit Scoring: If the historical training data used to train AI systems contains inherent biases against specific demographic groups, these AI systems may unjustly deny credit to individuals from these groups or offer them less favorable terms. This not only affects individual opportunities but also reinforces systemic disparities within the financial system.
- Loan Approvals: When bias is present in loan approval algorithms, it can lead to certain populations being systematically disadvantaged, thereby perpetuating existing economic inequalities. This happens because the algorithms, relying on biased data, may make decisions that unfairly favor certain groups over others, hindering equitable access to financial resources and opportunities for economic advancement.
3. Privacy and Data Security
The ethical use of customer data is paramount in AI deployment. Financial institutions must balance the need for personalization with the imperative to protect customer privacy.
Ethical Use of Customer Data
Financial institutions should adopt robust data governance practices to ensure that customer data is used ethically and securely. This includes obtaining explicit consent for data use and implementing stringent data protection measures.
Balancing Personalization and Privacy
While AI can enhance personalization in financial services, it is essential to strike a balance between offering tailored experiences and safeguarding customer privacy. Institutions must be transparent about data usage and provide customers with control over their personal information.
Bias in AI Systems
1. Sources of Bias
Bias in AI systems can originate from various sources, including historical data, data collection processes, and algorithmic design.
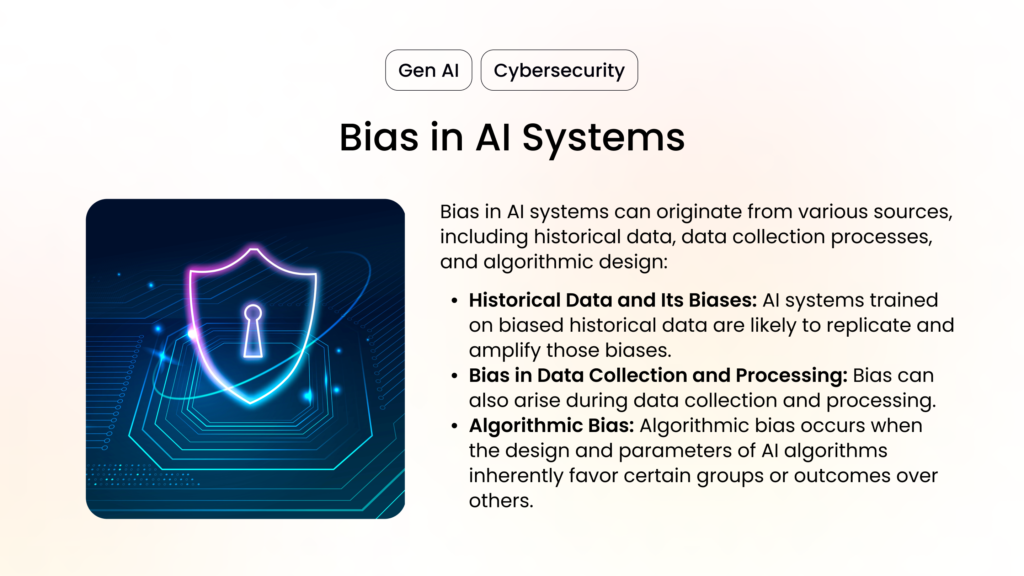
- Historical Data and Its Biases: AI systems trained on biased historical data are likely to replicate and amplify those biases. For example, if past data contains discriminatory patterns, the AI will learn and perpetuate these biases in its predictions and decisions.
- Bias in Data Collection and Processing: Bias can also arise during data collection and processing. Incomplete or skewed data sets can lead to inaccurate and biased AI outcomes.
- Algorithmic Bias: Algorithmic bias occurs when the design and parameters of AI algorithms inherently favor certain groups or outcomes over others. This can happen unintentionally but can have significant ethical implications.
2. Impact of Bias
The presence of bias in AI systems can lead to discriminatory financial outcomes and long-term consequences for underserved communities.
Discriminatory Financial Outcomes
- Credit Decisions: Biased AI systems can result in unfair credit scoring and loan approval processes, disproportionately affecting certain demographic groups.
- Investment: AI-driven investment strategies may inadvertently favor specific industries, regions, or demographics, reinforcing economic disparities.
- Customer Service: AI-powered customer service applications, such as chatbots, can exhibit biases in interactions, leading to unequal treatment based on demographic information.
Long-Term Consequences for Underserved Communities
Bias in AI systems can exacerbate existing inequalities, limiting access to financial services and opportunities for underserved communities. This can have lasting social and economic impacts.
Mitigating Bias and Ethical Problems
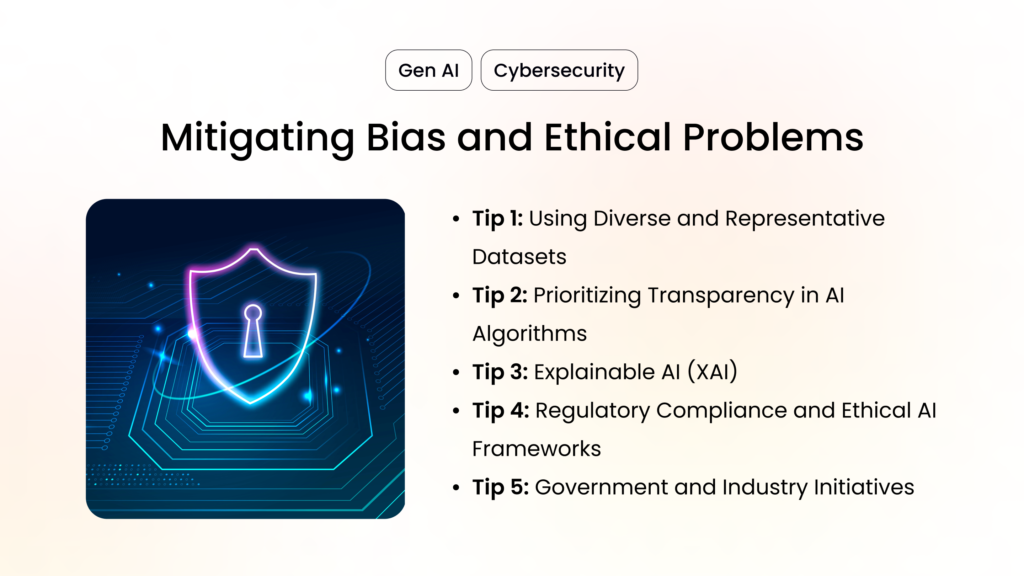
Using Diverse and Representative Datasets
To mitigate bias, financial institutions must use diverse and representative datasets that reflect the population as a whole. Actively seeking out underrepresented groups and including their data in training sets can help ensure fairer AI outcomes.
Prioritizing Transparency in AI Algorithms
Transparency in AI algorithms is essential for regulatory compliance and building consumer trust. Financial institutions should document and explain the decision-making processes of their AI systems, allowing for scrutiny and identification of potential biases.
Explainable AI (XAI)
Explainable AI (XAI) techniques can help demystify how AI algorithms generate specific outcomes. This is particularly important for providing understandable explanations to individuals affected by AI-driven decisions, such as adverse credit determinations.
Regulatory Compliance and Ethical AI Frameworks
Financial institutions must adopt ethical AI frameworks that prioritize fairness, accountability, and transparency. This includes:
- Regular Monitoring and Auditing: Continuously monitoring and auditing AI algorithms to identify and address biases.
- Clear Guidelines and Policies: Establishing company-wide guidelines and policies for ethical AI use, involving stakeholders in their creation.
- Regulatory Alignment: Ensuring AI systems comply with existing regulations and guidelines, such as those against credit discrimination and requirements for explaining adverse decisions.
Government and Industry Initiatives
Government initiatives, such as the White House’s executive order on fairness and avoiding bias, can provide guidelines for ethical AI use. Financial institutions should stay informed about evolving regulations and best practices to ensure their AI systems meet ethical standards.
Conclusion
AI has the potential to transform the finance and banking sectors, offering unprecedented opportunities for efficiency, personalization, and innovation. However, the ethical concerns and biases associated with AI deployment must be addressed to ensure fair and transparent outcomes.
By using diverse datasets, prioritizing transparency, adopting explainable AI techniques, and aligning with regulatory and ethical frameworks, financial institutions can mitigate bias and uphold ethical standards in their AI systems. This approach not only builds consumer trust but also positions institutions for long-term success in an increasingly AI-driven world.
For financial professionals, tech enthusiasts, CFOs, startup leaders, and company leaders, understanding and addressing AI ethics and bias is crucial to harnessing the full potential of AI in finance and banking. By taking proactive steps to ensure ethical AI use, we can create a more equitable and inclusive financial landscape.
Ready to dive deeper into AI ethics and innovation? Explore Innovators Hub Asia’s resources and insights to stay ahead in the evolving world of AI in finance.